What Machine Learning Is Not
Machine learning is not an AI. Well, you might be surprised because AI and machine learning are terms which are often used interchangeably in many articles, blog posts etc… But the truth is machine learning is “only” a subfield of a much broader field of Artificial Intelligence. Based on one of the most popular textbooks about artificial intelligence: “Artificial Intelligence: A Modern Approach (AIMA)” I created a picture, which presents this fact.
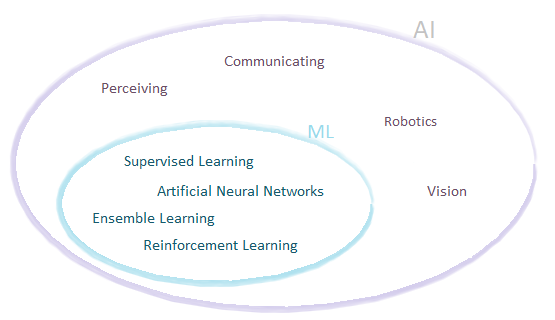
Machine learning is a very important part of AI, but only part of it. This should not be surprising because after all, we can say that AI is about agents acting rationally in the environment. To act rationally you need much more than only an ability to extract patterns from data. For example, you need to receive perceptions, to build knowledge about the world, be able to reason rationally, be able to act — machine learning is only a part of this great journey.
What Is Machine Learning?
So what part of AI is machine learning? There are several various definitions, but in its most distilled form, machine learning is to generalize beyond the data that was used to train the model.
It is similar to an induction method of reasoning: some general patterns are recognized based on some specific observations. However regarding the induction number of observations, there are hundreds, or even thousands at most. In addition, the reasoning agent is a human whereas in machine learning the number of training data could easily be millions where the reasoning agent is an algorithm.
How can we generalize the data and how do those algorithms work? Some most used machine learning approaches are mentioned below.
Training Machine Learning Algorithms
Supervised Learning
Supervised learning means that we train the model with data that contains inputs, which is correctly labeled. So for example, if we want to create a model that predicts housing prices then in addition to input data (e.g floor area, location, number of rooms, number of floors, etc…) we also have a price of each house. Then after training a model with enough samples (each sample represents a house) we expect that our model will be able to generalize, find correlations between input vectors and output (price) and correctly predict the price for houses from outside of the training set. The most popular algorithms used for supervised learning are mentioned below:
Linear regression
This is a standard, highly interpretable method for regression - which is modeling relationships between input variables which are independent of each other and depending on the input output variables. For example, this could be just a linear dependency between floor area and house price, easily representable on 2D space.
A business case might be to understand product-sales drivers like price, competitors price, quality, etc…
Logistic regression
This model is similar to linear regression but is used for a classification task. Classification means that we expect binary value as output. So the model predicts that something is either true or not. For example, in our case, we would like to know whether a house will be sold in the next 6 months, the output is either yes or no.
Another business case might be a decision whether a loan will be repaid or not.
Decision trees
A decision tree is a highly interpretable model that can solve both classification and regression problems. It is highly interpretable because it is represented as a tree that splits data values at each branch depending on some features. In each leaf of the tree, there is an output value.
A business case in our house example would be to recognize which features are most important for determining house price. Another case might be to understand product features that make it most likely to buy.
Random forest
This method is an example of ensemble learning. Its result is a combination of many various models of the same type. In the case of a random forest, it is easy to guess that the type of the model is, of course, a decision tree. Random forest improves the accuracy over decision tree by averaging the results from running the method multiple times. However, we are losing the high interpretability of simple decision tree.
A business case might be for example to predict power usage in an electrical grid.
Naive Bayes
This is a classification technique. It uses Bayes theorem to calculate the probability of events based on the knowledge of factors that might affect that event. It is a rather simple technique but for text categorization, it is competitive with more advanced approaches.
A typical business case is to categorize emails as spam or not based on occurrences of words in the text.
Support vector machines (SVMs)
This technique is usually used for classification but can also be applied to regression problems. This model represents training input data as points in space and tries to find a gap between categories that is as wide as possible. Then when a test vector is being checked it falls to one side of this wide gap and this side represents the result.
A nice business case might be to determine whether a user is likely to click on the ad or not.
Artificial Neural networks (ANNs)
Neural networks deserve separate text on their own, here are a few words to explain how they work. Neural networks try to mimic the way neural connections in the human brain work. They usually contain a few layers of neurons. Each neuron from one layer is connected to some neurons from the next layer. The first layer represents the input vector, and the last layer represents the output.
Neural networks are good at representing complicated non-linear dependencies between the input and output. They have been known since the 1950’s but until about 2000s we did not have enough computing power to make them work for non-trivial examples. The huge improvement in the field was also possible by rediscovery of the backpropagation algorithm in the 1980s. There are different kinds of neural networks: standard neural networks, convolutional neural networks, recurrent neural networks, however, I will not go into details of their specifics here.
Possible use cases are broad, they can solve all of the use cases mentioned in other models. Fine-tuned by an expert they can achieve much better results than models described previously. One of the typical cases is handwritten text recognition. This was an introduction of neural networks to make this task feasible for industry and reduce users usage.
Unsupervised learning
When we don’t have labeled training data but still want to find patterns in it we talk about unsupervised learning. Unsupervised learning algorithms try to infer structure from given data, most commonly they gather the training data into clusters (in multidimensional space). Then after training such a model, we can check to which category new input data belongs. For example, this seems as a good method for segmenting the customers according to different parameters we can gather about them. Some of the unsupervised algorithms are listed below:
K-means clustering
This algorithm clusters the data in a k separate groups (thus the name of the algorithm). Each group contains the input data vectors that are nearest to themselves in d-dimensional space where d is the number of attributes in each vector.
The business case would be for example to find groups of houses that are somehow similar to themselves according to parameters such as price, price per square meter, city, location, number of floors, etc… Another obvious business case is one mentioned earlier for segmenting customers into different groups to better target each group with marketing campaigns.
Hierarchical clustering
This algorithm creates a hierarchical classification tree where each node in the tree represents a group. Subnodes of a node represents a further split of the node group.
In a typical use case, you can cluster your customers into more and more detailed groups. As those groups are represented as hierarchy, you can for example, target marketing campaign to a group represented by a node that is on any level of the tree depending on your specific needs.
Recommender systems
Recommender systems are not a separate technique. It is rather that those systems usually use some clustering algorithms to identify similar groups for which similar things should be recommended.
A use case might be to recommend which movies a user should watch using user similarity to other users.
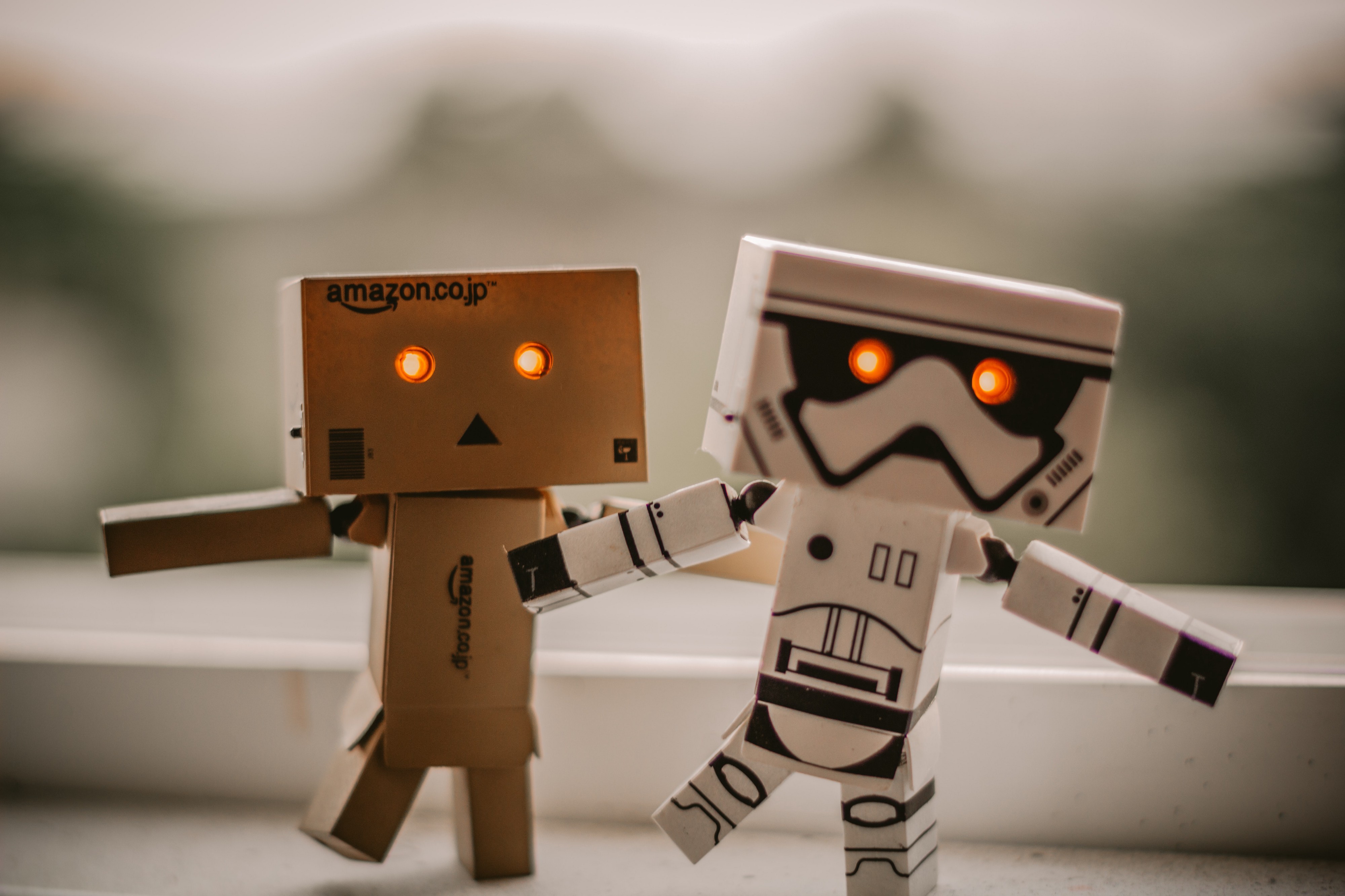
Reinforcement learning
Reinforcement learning is used when there is not enough data to learn or a program needs to interact with the environment to receive feedback about the value of its actions. During its lifetime reinforcement learning algorithm tries to maximize the reward it receives for its actions.
Some examples of use cases might be the optimization of trading strategy (receiving constant feedback about the rewards of previous decisions/actions). Another case could be to balance the load of electricity grids (also learning over time which actions maximize the balance of the grid).
How to Approach Machine Learning
As you can see machine learning is hard. It is easy of course just to use some predefined models, but in reality, we need to be able to solve a specific problem for a client. Such specific problem can be solved by a model trained to solve it. However, it requires a machine learning professional to do it properly.
According to some estimates, there are no more than 10k machine learning developers on earth capable to solve harder problems. Probably almost every software company would like to hire such a professional. There are at least 100k software companies in the world (we don’t have exact numbers but we have an estimate for a number of software developers and it is 21 million in 2018, so 100k software companies seems as a very conservative estimate).
The conclusion is that it is hardly possible to hire such machine learning professional. It seems that the only way left is to cooperate with people leaving universities after machine learning courses and/or people trying to change their career path and become machine learning experts.
This means that your company needs to be prepared, meaning that it will take time to grow and encourage such individuals to be experts and that failures may occur. However, the field is exciting and potential machine learning know-how gathered is of tremendous value. So good luck in nurturing a machine learning team and solving exciting machine learning problems in your organization.

Related articles
Supporting companies in becoming category leaders. We deliver full-cycle solutions for businesses of all sizes.